Unplanned downtime is costly in many aspects within manufacturing. It reduces delivery capability, customer satisfaction, and increases overall costs. So which maintenance approach should we use to reduce the frequency of downtime?
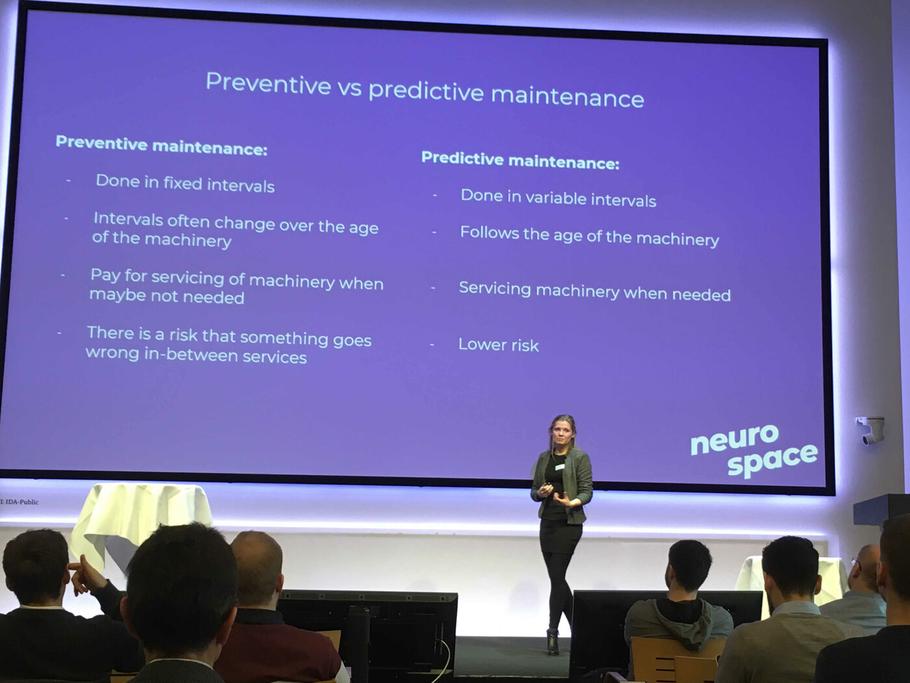
At IDA industry 4.0 conference, we talked about how to use machine learning for predicting unplanned downtime to reduce overall costs.
How costly is unplanned downtime?
Let’s look at what surveys says about unplanned downtime. The total cost of unplanned downtime depends on what your company produces, the cost of raw material, and frequency of machinery downtime. It can therefore vary what an hour of downtime costs. It additionally depends on the given segment, where an hour of downtime of cruise ships or airplanes is expensive, while the cost of unplanned downtime for producing chocolate might be lower, yet significant.
A field survey of 450 companies across different segments such as manufacturing, medical, oil and gas, energy and utility, distribution, telecoms, and logistics and transportation found that:
⋅⋅⋅ “82 percent of companies that have experienced unplanned downtime over the past three years outages lasted an average of 4 hours and cost an average of $2 million”
⋅⋅⋅ “Unplanned downtime results in loss of customer trust and productivity - 46 percent could not deliver services to customers”
⋅⋅⋅ “70 % of companies lack completely awareness of when equipment assets are due for maintenance or upgrade”
Another survey from BHGE, 2016 on offshore oil and gas finds that using data and monitoring for maintenance is the least costly approach when compared with planned and reactive.
Disclaimer: the study does not clearly present what is meant with annual unplanned downtime days, what the value is for each maintenance approach, or how this is calculated. Therefore, we will disregard this in this blog post
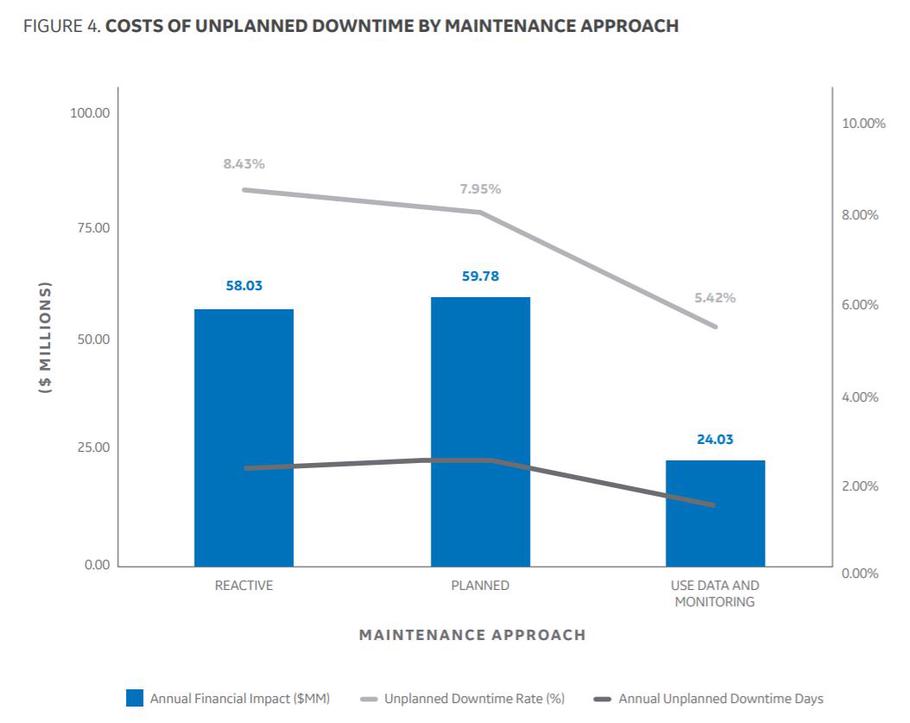
Cost of different maintenance approaches
Reactive: When something is broken - fix it-approach
Using the reactive approach in manufacturing means that the production runs until something breaks. There are no maintenance involved until the machine breaks which means that your factory must be equipped to handle downtime (e.g. if you have a larger safety stock or produces after the “made-to-stock”-approach).
In their survey, the reactive method had an annual cost of 58 million dollars, with an average unplanned downtime of 8.43 % (see figure above) or an uptime equal to 91.57%.
If a factory runs 24/7/365 operations this amounts to 30 days and 18 hours of downtime or what equals to a total month of production!
Planned (preventive): scheduled maintenance
In planned maintenance, a company most often has an agreement to get the machinery serviced once a month, once a quarter, or once a year to reduce the risk of downtime. However, this leaves a gap in between two service periods, where machinery can be overheating or screws can get loose, causing a machinery breakdown and thereby unplanned downtime. Additionally, you might pay for servicing the machinery more often then needed.
In this survey, the planned method had a cost of almost 60 million dollars, with an average unplanned downtime of 7.95 % (see figure above) or an uptime of 92.05%
Data and monitoring (predictive): Using data to know whenever something within the machinery changes
Predictive maintenance uses data from machinery, similar to taking the temperature of the human being, to tell whether the machinery is well or “sick”. When using data from machinery, you will always be aware of how well the machinery is performing.
You can get a warning about machinery breakdown days or weeks before it occurs, and thereby plan your maintenance outside of scheduled operating time.
Additionally, we can create an extra model, that is triggered by “sick data”, that can tell you the causation for machinery breakdown. On that way, you will know immediately where to put your focus on, when maintaining the machine.
You will not only minimize the cost on servicing the machine to when needed, you will also minimize the time it takes to service the given machinery.
In this survey, the predictive method had a cost of 24 million dollars, or 60 % less than the planned method and 58.6 % less than the reactive method. And had an average unplanned downtime of 5.82 % or an uptime of 94.28%.
“The unplanned downtime for respondents using more predictive data- and condition-based monitoring approaches is 36% lower than respondents using a more reactive approach.”
How to get started?
So how do you go from using reactive or planned maintenance to predictive maintenance? It all starts and end with your data. We need to collect data from the machines. Good predictions for future breakdowns could be found in for instance the temperature, rotational speed, vibrations, and sound as we found in Predicting hard drive failure with machine learning.
The data is collected with different sensors attached to your machinery, and stored in a data warehouse or data lake. To make good predictions there is a need for having data from the past to compared it with current data. The data must be labeled as “healthy” data or “sick” data. It is crucial for your machine learning model, that you have data available from both cases! It is not possible for a machine learning model to find patterns, if both cases are not represented in the dataset.
When you have enough data, a machine learning model can be created which can be used for predicting future events! Kimberlite study found that a 1 % unplanned downtime in the oil and gas industry (or 3.65 days of downtime) can provide an extra cost of $5.037 million annually. This indicates that if we can create a model that is just 1 % better than your currently best practice, the costs saved will be more than visual on your bottom line. The 60% lower annual financial impact by using predictive maintenance could according to BHGE result in $34 million in earnings on average per year.
But you must start gathering relevant data today!
According to landing.ai an AI transformation in your company can take up to 2 - 3 years, but within 6 - 12 months you can see initial concrete results if you start the right way!
If you start today it can improve your delivery capability, reduced costs, and increased uptime, and help your company reach its Key Performance Indicators (KPIs).
// Maria Hvid, Machine Learning Engineer @ neurospace