With the introduction to Big Data and later Industry 4.0, an increased focus has arisen on the usage of data in the industry. Even though industry 4.0 still rises confusion to what exactly it is and what it is not, one thing we can agree on is that it involves data.
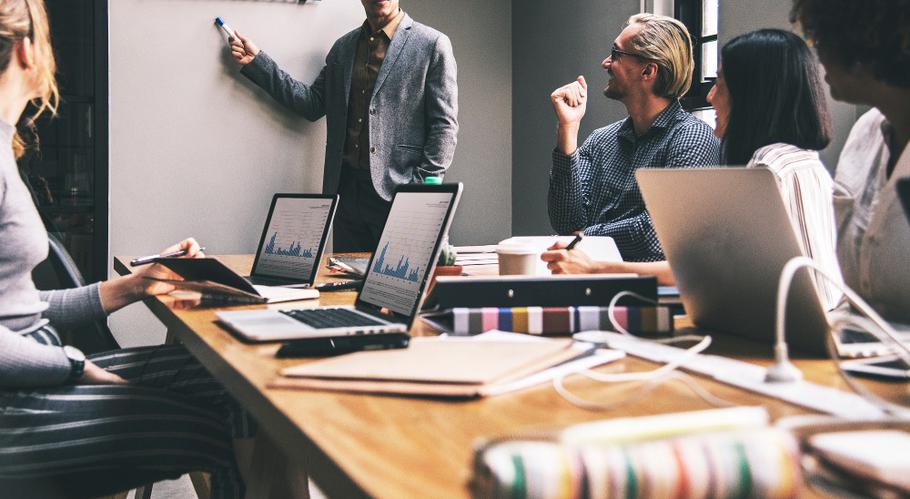
Big data has its focus on volume, variety, and velocity in the data. With machine learning, an increased focus has been placed on the quality and reliability of the data collected. These parameters have later been applied to big data with the introduction to veracity and value.
Data has the potential to develop a new business area of which you can gain critical performance insight, and a competitive edge.
Based on your company’s historical data, machine learning algorithms can discover complex patterns that can be used to:
- Automate processes
- Take data-driven decisions
- Predict future events
Additionally, it can help detecting , like we do in predictive maintenance, helping companies improve uptime and availability in Overall Equipment Effectiveness (OEE) . Machine learning and statistics can predict future sales, helping determining how many products to produce to meet customer demand, and how many raw materials you need to have on stock to be able to produce it. The possibilities are endless, and only limited by your imagination - and what cannot logically be solved.
With industry 4.0 and machine learning, titles like Chief Data Officer (CDO), Data Scientist, and Machine Learning Engineer have been a more common to see even in the manufacturing industry. Companies have to consider their strategy towards data - not only to know what data to collect, but also to identify the purpose of collecting data.
Data Strategy
If you receive Ingeniøren or Maskinmesteren’s newsmail you cannot avoid hearing about machine learning and artificial intelligence (AI). At least five pages each week contains content about either ML, AI, big data, or Industry 4.0. Yet, still only few manufacturing companies have decided to seek the opportunities of ML in their company [1].
The data strategy should be defined by the Board of Directors and the leadership team. Therefore, the Board of Directors and leaders within the organization need to know what benefits can come from ML, and what challenges might lay ahead. To be able to do that, you must know what machine learning is and be able to define ML pilot projects.
There is no need to wait years before you can get value from your ML projects. You can start from scratch and get value within months. That is why neurospace believes in: Be ambitious, start small, and gain value fast.
Be ambitious
Follow the Value Stream Mapping (VSM) approach; draw your current production with the mean failure rate, average overtime costs, costs on defective and discarded products. When you have drawn the current VSM, it is time to be ambitious! How do you want the future VSM to look like? How many breakdowns are acceptable, how many defective and discarded products are okay, and how much money on overtime are you willing to pay? This can now be defined as Key Performance Indicators (KPIs) - e.g. by using OEE with an objective to have an availability of 97% or by having a KPI with the objective to have an uptime of 97%.
When you have drawn the future VSM, it is time to discuss the how. Start planning pilot projects, not in details but more in terms of what the pilot project should seek to prove and what value to find.
Start small
You do not have to collect data from your machines in years before you can start your pilot project. Additionally, you do not need to invest in sensors on all your machines at first. Start with one machine, proof the concept, and scale. Thereby, you have reduced costs on the pilot project and have a well-defined small project that can be done quickly. Additionally, you can get value fast!
A pilot project could be defined as: “to investigate the potential for predicting future breakdowns in our production settings”. The best place to start might be your bottleneck, or maybe an old machine of which maintenance is expensive or parts are difficult to buy.
When you have seen the potential, you can scale the solution to the entire company.
Get value fast
By defining pilot projects, and testing these on one setting, you can reduce the total costs on the project, as well as get value within months instead of years. This enables the organization to learn fast because of a fast feedback loop. Furthermore, it help people in your organization see the value and understand machine learning. This is important to create momentum for when you want to scale the solution.
The data in data strategy
When deciding on pilot projects it is important to know if the data produced by equipment in your manufacturing is actually yours? Often we see robotic cells and other machinery which delivers all its data to cloud services owned by the producers. Then the question becomes do you or the producer of the equipment own the data? An easy test is to see if you can get the data in a raw format, no pretty graphs, just raw data. If you can then you can utilize this data for pilot projects within your production - if not we believe you should do something about it. As mentioned in Why we started an ML company part 2 we believe ml has the potential to change the world including your production line and data is the key to unlocking this potential.
// Maria Hvid, Machine Learning Engineer @ neurospace
References
[1] Stig Yding Sørensen (2018) Kunstig Intelligens på vej i danske virksomheder. Teknologisk Institut.