Digitization is a word that is mentioned in most meeting rooms. We have met many companies that have a 2025 goal of digitizing parts of their business. But what exactly is digitization? What are the benefits of becoming more digital? When is it satisfying and how can one kickstart the process? Is it important to your business at all?
What is Digitization?
Digitization is when we move something from the analog world into the digital world e.g. business processes. A concrete example could be the random sampling that is done in most companies. Some still do it analog on paper but more and more companies are shifting quality control over so it becomes digital.
Degrees of Digitization
Can digitization be qualified? Or rather, can we use a particular mindset to better understand the nuances of digitization? We know that some companies are more digitized than others - to describe this we have tried to qualify digitization.
We believe that there are benefits to seeing digitization as a journey where we start small and end up with what some will think is the holy grail. However, it is important to understand that travel is rarely linear, but we will return to that shortly.
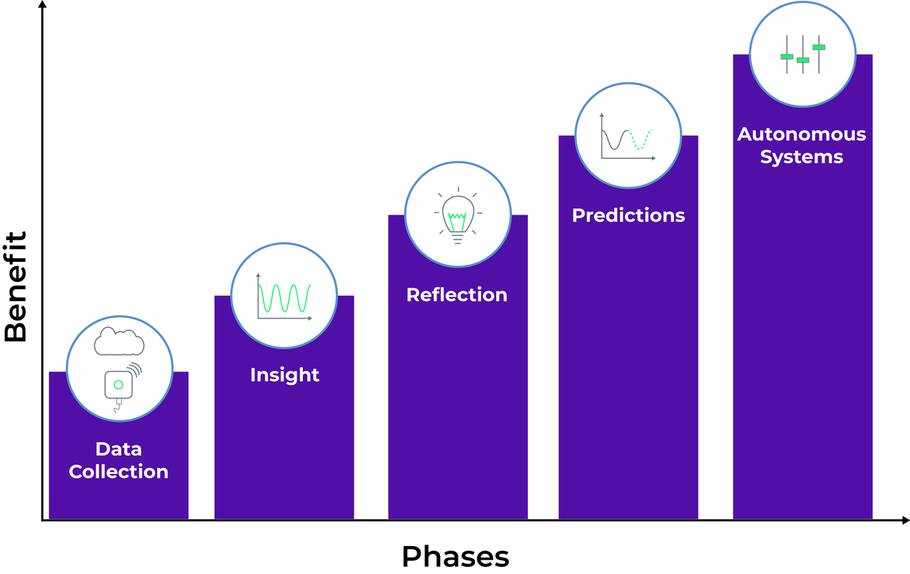
As visualized on the image above, the degree of digitization can be explained with five different levels:
- Data Collection
- Insight
- Reflection
- Predcitions
- Autonomous systems
A headline is good but details are better so let’s go through all the levels together.
Data Collection
Data collection is, as the name suggests, about beginning to collect data digitally. It could be as in our previous example of sample quality control that we pick it up in a system digitally instead of doing it analog. Collecting data allows us to compare it over time and make general statistics on our numbers. It can also provide the opportunity for other departments that might be interested in the quality to access the data. But it is important to understand that data collection without using it does not provide value in itself and that even small amounts of data can be very valuable if used properly. Many of the companies we help have challenges with data collection precisely because it involves collecting data with sensors, which requires different competences such as process, IT and Internet of Things (IoT) knowledge to get data collected from the processes. Therefore, it can be good to get involved with a partner who has a sense of not only IT but also to collect data from analog processes. At the same time, we unfortunately see that many people try to put all data in their relational databases, which is often suboptimal when dealing with time series or unstructured data such as video and images. The data collection can be seen as the infrastructure that enables the rest of the journey and building a good highway providing fertile soil for a successfull digitization journey.
Insight
Once we have data available digitally, we can start using it to create insight. Eg. that every Friday at 12.00 the quality is always low in our samples. Remember here that correlation is not causality. Therefore, we need to investigate more deeply what causes the dive in our quality on Friday. What we often do with you is try to visualize the data in different ways depending on what we are looking for. An example could be that we try to find fluctuations in the quality, what we call an anomaly, and here we could use visualizations such as swarmplots.
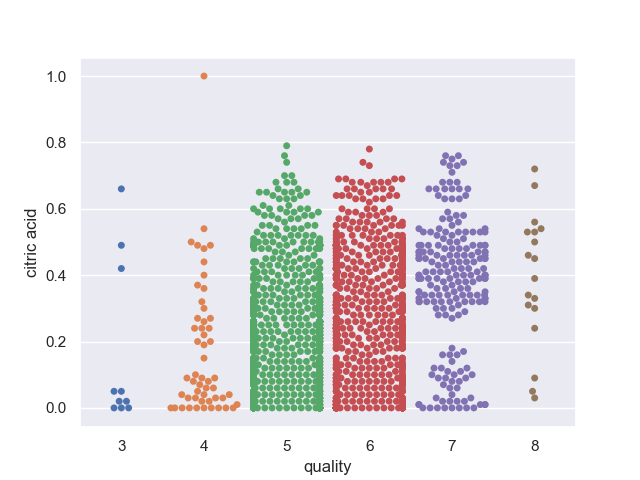
Here it will be clear to see the values that are outside the normal range. Insight is about understanding that we have a challenge that we want to solve, the reflection is when we try to understand why it happens.
Reflection
Once we have gained an insight, we reflect on why the situation arises on Friday and seek a causal explanation, also called causality. It may be that by talking to the teams on our production lines we find out that the handover from the night to day team is suboptimal and therefore the quality drops drastically, which we only see at 12.00 as this is where we make our sample. Such a reflection on why our data shows that quality is declining can lead to us being able to improve our processes and working methods so that we improve our business.
Predictions
Once we have the data collection in place and have some understanding of our data, we can start making predictions about when the quality will drop. Predictions give us the huge advantage that we can be proactive in our decision instead of always reacting on the back burner when something has gone wrong. In our example of sample control, we could change our process so that we collect data at a higher frequency, e.g. every 5 min or every product. When we do this we can start looking for the patterns that arise before the quality starts to slip. At the same time, we can detect poor quality early in the process and e.g. start sorting out bad or defective products from so we do not waste time on them and that they do not end up out at the customers who will fill out a complaint. If we can find these patterns, we can get predictions where it is possible to directly influence the process for the operators so that the quality does not fall outside the specification.
Autonomous systems
Sometimes autonomous systems are called the holy grail of digitization. Autonomous systems themselves can, based on data, understand the process and correct if predictions say something will go wrong or degrade in the future. The reason why autonomous systems are the last step on the journey is that it requires good control of the processes, that the data collection and predictions are to be trusted, and that we know what corrections a human operator would make.
Is digitization a staircase or a continuous process?
Learning ladders are good for explaining a concept, but the real world is more complex. It also means that the diagram with the digitization ladder you have seen so far is not how we look at digitization in neurospace.
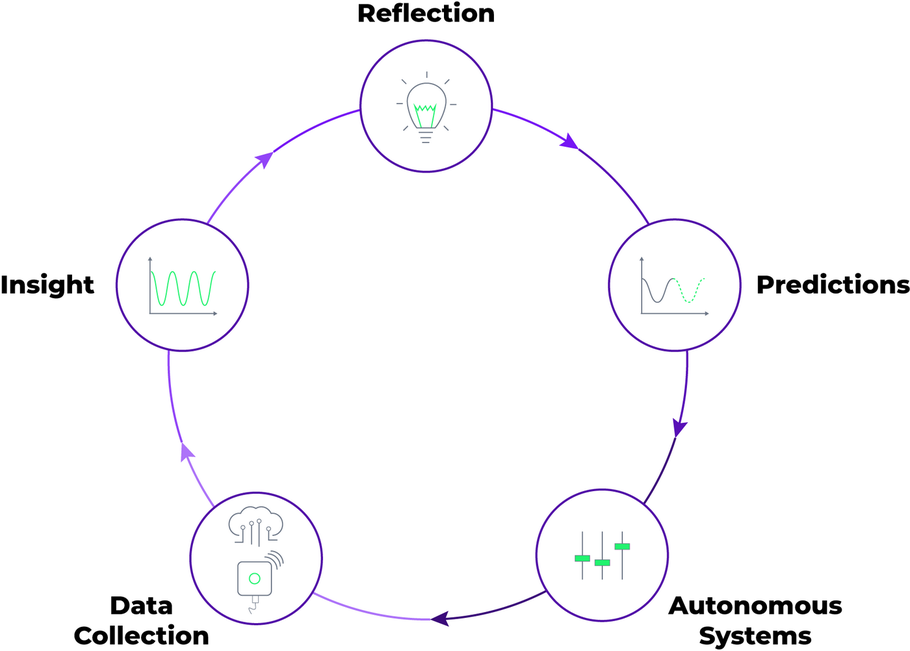
We see digitization as a continuous process in all companies. Through our work with our customers, we have seen two approaches within digitization:
- Select an area and create improvements by running an intensive pilot project
- Try to raise the level throughout the company by first starting with data collection
Our experience is that the value comes faster and becomes much more concrete if the company selects an area and develops a pilot project. A challenge with the 2nd approach is that it quickly becomes very abstract when it comes to digitization and data collection across the entire company because you cross many different work areas and workflows. Therefore, we recommend everyone to see digitization as a continuous process and be very specific with the value desired by making a digitization in a specific area.
Conclusion
Digitization becomes easier if we start small and focus on one area. Digitization can include everything from giving the right people access to the right data and up to creating autonomous systems that can help automate manual processes. At the same time, we must not be technology-scared when we start digitizing an area. Eg. We have proven several times that artificial intelligence (AI) can be quickly implemented to create predictions that can create business value and at the same time make processes more digital and automated. If you set this as a goal you will get here faster instead of getting stuck in implementing data collection across the company. Therefore, our recommendation is that you are ambitious with your digitization plan. Aim for digitization measures that can eliminate unnecessary work by making predictions. This means that the people we have in our companies can focus on what creates value for the company and the customers.
If you want to know more about how we help our customers get started on their digitization journeys or support a digitization, you can read more about our AI Camp where we focus on creating value quickly by creating an intensive process together as partners.
// Rasmus Steiniche, CEO @ neurospace