Nordic Sugar in Nakskov has identified an optimization opportunity by adopting a more data-driven approach to their crystallization equipment. As part of the project, Nordic Sugar and Neurospace formed a task force to explore the possibility of using machine learning to provide data-based feedback to the operator and thereby reduce energy consumption in production.
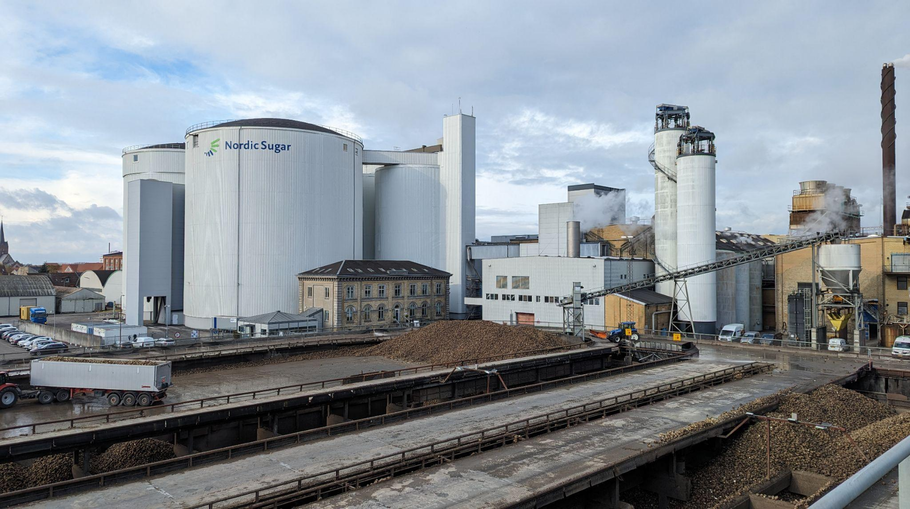
Facts about the company
Nordic Sugar A/S was founded back in 1883 and is today part of the Nordzucker group. The factory plays a central role in advancing a greener agenda in sugar production in Europe and has a strategic goal of reducing its emissions by 70% by 2030.
Summary
Basic knowledge about crystallization equipment
“Crystallization equipment in sugar production is used to concentrate the sugar solution under pressure and temperature. The vacuum allows the water in the sugar solution to evaporate at lower temperatures, so the sugar is not damaged. The resulting concentrated sugar solution then undergoes the crystallization process, where sugar crystals are formed.
The steam used in the crystallization equipment is recycled from previous production stages where higher temperature steam is needed. Therefore, the energy needs of the crystallization equipment are covered by recycled residual heat from evaporation (stage 4 and stage 5) at the factory. The steam can no longer be used, as the cooling of the steam itself is used to create the vacuum inside the equipment.
By reusing steam from previous processes, the factory’s overall energy efficiency is increased, thus strengthening Nordic Sugar’s goal of greener sugar production”
The Project
Nordic Sugar’s production runs 24/7 from the first beet being dug up to the last one being turned into sugar. This period is also described as a “campaign.” Production should be seen as a single-line system, and each step in the production has a cycle time that must be adhered to. When production runs around the clock, it is essential that all cycle times are met to ensure that there are no disruptions in production.
Operators in production have identified untapped potential in the fact that the cycle time around the crystallization equipment was often not fully utilized. This is because the sugar solution often boils faster than the 3-hour cycle time for A-product. In practice, this meant that too much stage 4 steam (high energy) was used, and there was potential to use more stage 5 steam (low energy) to fully utilize the cycle time with lower power.
Nordic Sugar and Neurospace held a kickoff meeting where the challenge was highlighted, and knowledge sharing was primarily aimed towards Neurospace to ensure that the necessary domain knowledge was present to be able to start working on the project. There was no single answer to how the task should be solved. However, it turned out that it was important to involve domain experts, who in this case were the operators and relevant technicians. The experts could provide insight into how production was carried out and which factors were important to consider. Based on this knowledge and data from previous production campaigns, a machine learning model was developed to predict when it was necessary to switch from stage 5 steam to stage 4 steam.
Results
What we anticipated and estimated based on the pilot project was | ||
---|---|---|
Increase in stage 5 steam | 7% | 43% |
Power requirement | 6.349 kWh | 39.004 kWh |
Diesel | 634 liter | 3897 liter |
CO2 | 1690 kg | 10.385 kg |
The test campaign took place over 34 days and the ML model was tested on 2 vacuum pans.
The results from the test campaign was | ||
---|---|---|
Increase in stage 5 steam | 40% | |
Diesel | 3000+ liters |
This result contains a bias because the process currently operates with a so-called “human in the loop.” This means that an operator still has to change the setpoints. The difference now is that they have a tool they can use to make a better and faster decision, based on the OWLS model’s predictions.
Specifically, operators have adjusted the setpoint in 80 out of 104 boils, whereas they had previously adjusted the setpoint in 30 out of 104 during the same period. Therefore, it can be concluded that the OWLS model has helped operators make better decisions more frequently, which is also reflected in the reduction in energy consumption.
If the model’s control of effect 5 steam consumption is fully automated in the long term, the setpoint will likely be changed more frequently. As a result, the gain from using the model will be even greater than what we have seen in the 34 days.
In addition to reducing energy consumption, the project has also had a positive impact on efficiency and steam balance at the factory.
The OWLS case should be seen as a dynamic solution, as the conditions in the vacuum pans depend on a wide range of factors such as the size, type, piping, and the general operation of the factory. Nordic Sugar chose to collaborate with Neurospace and to invest in a machine learning solution, because they had a clear expectation of the project’s potential and the factors that should be included in a static solution. At the same time, they had a lot of open questions and a clear desire for a method that can be reused across devices and factories.
Scaling Potential
The method developed during the OWLS project, trains very small ML models that answer some very concrete questions. The method can thereby be used on new vacuum pans, to train specialized models from scratch. It takes approximately one minute to train and search for the optimal hyperparameters for the models that produce the results described above.
In Neurospace we try to cut to the chase and have no ambitions of building large, general ML models, we call this “Lean Machine Learning”. In this way, the small investment that Nordic Sugar had put into the project, quickly resulted in great value, without using unnecessary energy on driving large models.