For a large Danish production company, we have investigated whether already existing data can create a basis for making remaining useful life estimations.
Facts about the company
This case is anonymous as there are patents involved and therefore the name of the company will not be mentioned. The company works with food production and employs approx. 500 employees and has a turnover of about DKK 2 billion. They are leaders in their industry and are known for their innovative approach.Summary
-
Current maintenance system can not separate preventive downtime and unplanned downtime - it is a necessity to be able to create good analyzes
-
The number of breakdowns was not as extensive as first assumed
-
Historical breakdowns lacked cause - it could not be verified whether breakdowns are due to wear and tear or sudden failures
-
The model created in the project was able to capture the few breakdowns that had a long life cycle
The company initially thought they had good control of their maintenance journal and that they had “lots” of breakdowns. However, it turned out through the project that the level of detail in the maintenance log varied greatly and that the number of breakdowns was less than first assumed. However, with the help of Neurospace, some breakdowns were labeled as unplanned and our Cohen library was used to create Remining Useful Life (RUL) models that captured those breakdowns with life cycles longer than 1.5 months.
The project
Through an AI Camp, we investigated whether historical breakdowns are sufficient to be able to predict the remaining useful life. Thus, we investigated not only whether there are sufficient unplanned breakdowns to be able to make a satisfactory Machine Learning model, but also whether the data quality is sufficient.
Data include sensor data such as pressure and temperature. Thus, high-frequency vibration measurements are not included in the available data set. The company also has a goal that the model should be able to predict the remaining useful life accurately approx. one month before the breakdown occurs. This enables the company to order spare parts and schedule maintenance into a 24/7 production, where the steam lock is a critical component.
When analyzing logged breakdowns in operation and the maintenance log, however, it can be verified that on average less than 1 month passes between logging. As it is not possible to verify whether these logs are scheduled downtime, unplanned downtime, or ongoing maintenance such as greasing the machine without downtime. When there doesn’t exist many breakdowns that last more than a month in the data, it is difficult for a Machine Learning model to learn the pattern. Therefore, the company has learned a lot about their data quality, own maintenance situation and data.
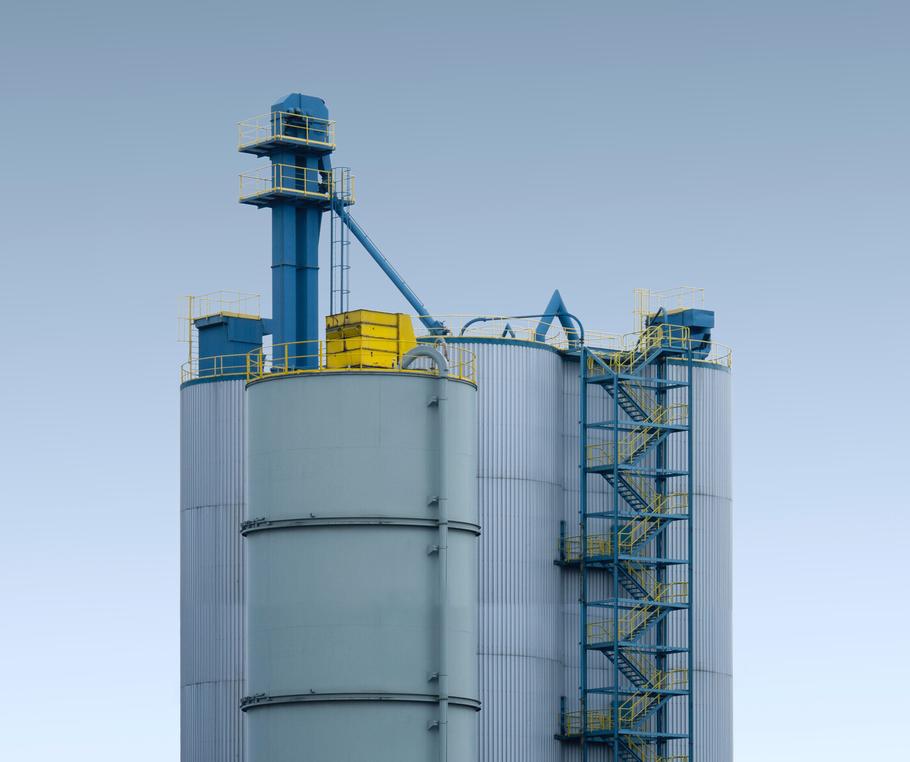
The Machine Learning model that was developed during the AI Camp captured the trend and breakdowns when the life cycle was longer than a month and a half. However, it had difficulty finding a connection when there was a very short interval between downtime and the correlation to the various components. This can be improved by using high frequency vibration sensors or ultrasound. Furthermore, the model was affected by preventive maintenance measures as these have a major impact on the remaining useful life. Together with Neurospace, the company has proven that there is potential in Predictive Maintenance for them, but that they need to change their maintenance procedure to get the full benefit.
Following this insight, they chose to run longer in between their scheduled maintenance and had no crashes. Which could indicate that over-maintenance is taking place and that there is a greater saving to be made.
Customer Value
The customer learned during the AI Camp that they need to be better at noting different types of breakdowns in their operation and maintenance log. A distinction must be made between when to perform preventive maintenance that does not affect operation, a planned shutdown, and an unplanned shutdown. Furthermore, they found that they may not have as many breakdowns as they initially thought, and many of the breakdowns that occur are actually sudden, and not caused by wear and tear.
The customer also discovered that there is potential for Predictive Maintenance in their production and that there is a business case, both in terms of over-maintenance but also better planning of maintenance to avoid downtime.
During the AI Camp, they also found that the vibration sensors purchased for other equipments collect data with too low a frequency to be able to use them for Predictive Maintenance.