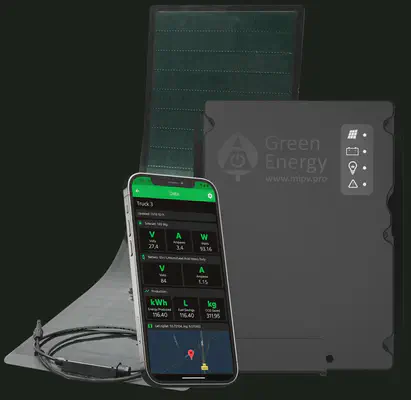
Google Cloud Scalable Data Platform - Green Energy
With a strong foundation for a data platform, you are able to create an effective and powerful interface where data becomes accessible to the team. This means they, at all time, can process analysis and extract relevant data for specific business cases.
In this way, agile processes are optimized, increasing the flexibility and efficiency in your company’s workflow. Choosing the right data platform increases the scalability of your data, and the architecture of the data can give your company better data governance.
At Neurospace, we work from a data mesh approach, where the architecture is built on both data sources, data infrastructure and domain experts. This approach bridges the gap between the operational and analytical parts of data collection.
A data platform can be a valuable asset for any business. By implementing a data platform, you can:
- improve your decision-making
- increase your efficiency
- enhance your innovation
- improve customer satisfaction
At Neurospace we recommend making the platform as code. It will increase the security surrounding the platform and make it more efficient, because of the possibility to always restore and see the history of the code. Beside that we encourage a ‘4 eyes’ principle, which means that changes must automatically be approved by at least two people to secure the quality of the code.
In Neurospace we think it’s important that the data platform is agile, which means that it should be able to work and interact across systems and departments. This is why we tailor data platforms, so that it will become efficient for the workflows in your company. An example of this could be excel, if it is important to extract data from the platform to excel it is important to make the data platform compliant.
A tailored platform can reduce cost and automate processes.
By simultaneously exposing data freshness and its lineage, transparency and trust in data are increased. By moving data ownership out into the business, it is possible to increase innovative thinking and each department will be able to build data products and train machine learning (AI) models themselves through this.
Whether you want to work with Databricks, Snowflake, BigQuery, Azure, or another cloud-based data platform, we are compatible with collaborating with your company to build a robust and scalable data platform. We offer expertise in designing, implementing, and managing data platforms that meet your specific needs and goals.
In our collaboration we strive to work with domain experts, which is crucial for how we develop the solution. We also strive to onboard and involve the employees as a running process throughout the development of the data platform.
Regardless of the technology you choose the platform to be build in, Neurospace will deliver a platform that is able to:
Big Data? No, Right Data!
Neurospace believes that data should have a strategic goal, and the size of the data should be the least important aspect of this goal.. It is the quality of the data that is important. This is why we use the ‘Right Data Framework’, meaning that we only retrieve data when it has a purpose. This strategic approach ensures:
Google Cloud Scalable Data Platform - Green Energy